Machine Learning Applications In Commodity Price Forecasting
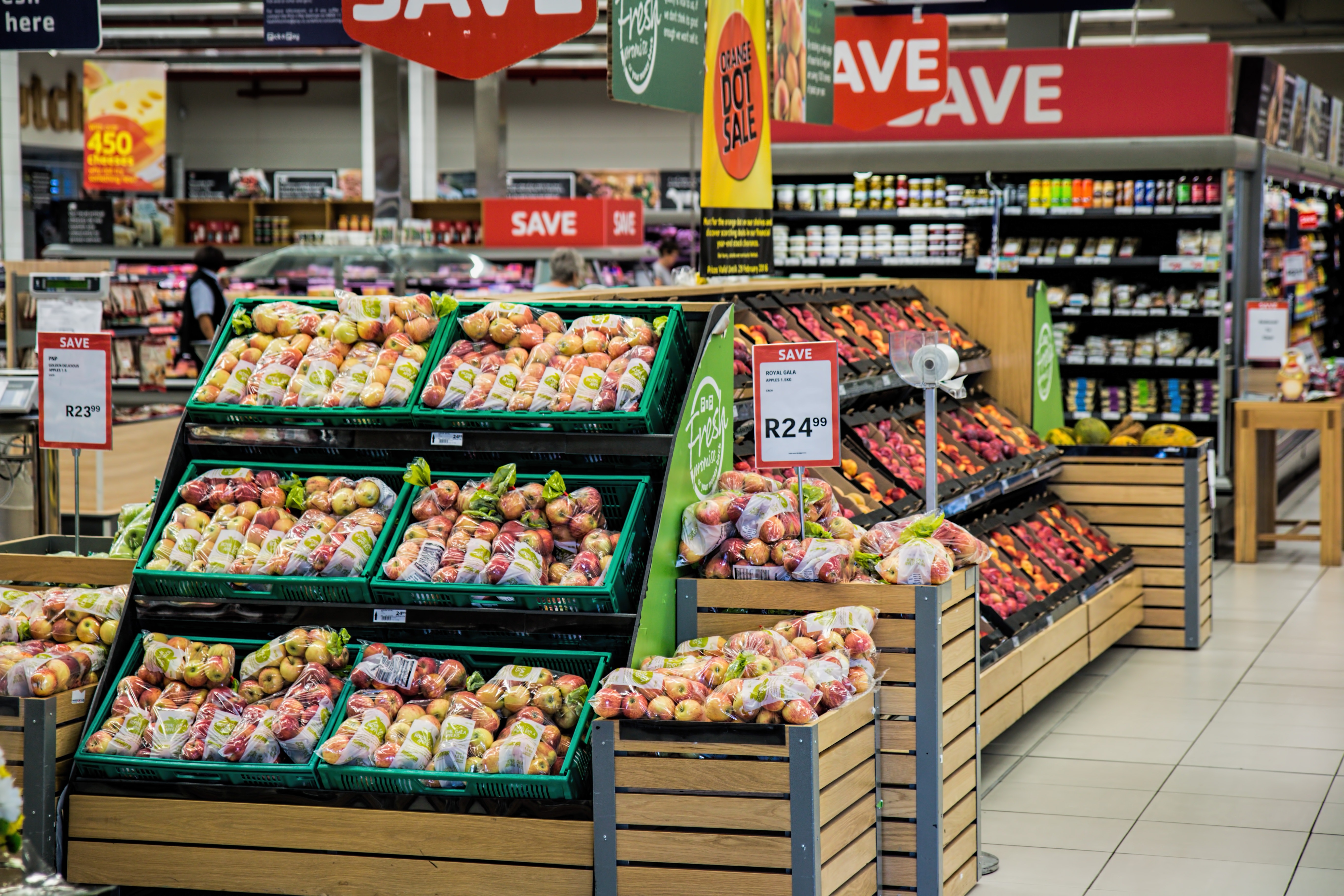
Forecasts of commodity prices are integral to today's trading and investing landscape. Nevertheless, these predictions are wrought with complexities. Enter the world of machine learning. Machine learning applications in commodity price forecasting are fast gaining traction, signaling an innovative phase of data-driven predictions. Before diving deep into this confluence, let's understand the separate elements more clearly.
Machine learning, at a basic level, involves ‘teaching’ computers to learn from data and make intelligent decisions. Meanwhile, commodity price forecasting involves predicting the future prices of commodities based on various factors. It's the juncture of these two disciplines where a revolutionary change is underway.
Commodity prices form the lifeblood of the global economy. These prices impact everything from the running of multinational corporations to the price consumers pay for their morning coffee. Effective forecasting methods are thus critically important to investors and organizations. With precision in their predictions, stakeholders can manage risks better, ensure supply-chain stability, and strategize investment moves confidently.
Traditional methods of commodity price predictions, such as statistical analysis and fundamental analysis, do have their place. They can provide a broad understanding of the market and macroeconomic conditions influencing price actions. Nonetheless, these methods often grapple with limitations—they, at times, struggle with large volumes of data, their analysis can be error-prone, and human biases may slip in.
Machine learning ushers in an exciting new era of data analysis and forecasting, presenting a promising alternative to these traditional methods. Machine learning systems can churn through vast quantities of data at lightning speed and extract useful insights. The most popular types can be grouped into supervised learning, where the systems learn from examples, and unsupervised learning, where the learning happens through the exploration of data patterns. The advantages of machine learning applications in commodity price forecasting are becoming increasingly manifest as it can eliminate many of the challenges associated with traditional methods.
Machine learning can recognize intricate patterns in commodity price data which the human eye would often miss. Prediction models, such as regression analysis and decision trees, can generate insights and trends directing towards more accurate price prediction. This sharp accuracy that machine learning can bring about has significant implications for those interested in price predictions, as it offers an edge in a highly competitive marketplace.
The future of machine learning in commodity price forecasting is beaming with potential. As technology continues to evolve and data becomes ever more accessible, machine learning's integration within this area is clear. Increased computational power along with data abundance will further help refine these predictive models. Moreover, the innovations around the corner, like better algorithms and more sophisticated models, promise more precise forecasting, making this union an area ripe for growth.
The amalgamation of machine learning and commodity price forecasting brilliantly encapsulates the evolution of technology within the finance sector. From providing more accurate forecasts to enhancing the efficacy of trading strategies, the impacts are manifold. It’s evident that machine learning has disrupted traditional methods and holds remarkable potential to transform commodity price forecasting even further.
Looking ahead, we can expect the intersection of machine learning and commodity price forecasting to go beyond its present applications. Creating an environment where data-driven decision making is the norm. Truly, machine learning applications in commodity price forecasting are not only shaping the present but also crafting an extraordinary future for the finance sector.
In conclusion, this confluence of machine learning and commodity price prediction represents a pioneering phase of data-centric prognosis. The innovative use of machine learning has significantly enhanced our ability to orchestrate commodity price predictions with higher precision. As this technology continues to evolve, displaying a symbiotic relationship with data, it’s destined to create a seismic shift in how forecasting is conducted in the financial realm. The future of commodity price prediction is highly likely to be dictated by machine learning applications. And investors who can harness the full potential of this trend will, no doubt, be leading the pack.
Author: Brett Hurll
Behind The Beans: Unpacking The Causes Of Cocoa Supply Shortages
The cocoa industry is currently facing significant challenges, with supply shortages disrupting the market. Understandin... Read more
Democratic Accusations Against Big Oil: Unveiling Climate Change Denial And Doublespeak
In recent years, the urgency of addressing climate change has become increasingly apparent, prompting scrutiny of indust... Read more
Archer Daniels Midland's Profitability Soars: A Beacon Of Strength In Global Agricultural Markets
Archer Daniels Midland (ADM) has recently made waves in the financial world by surpassing expectations with its stellar ... Read more
Exxon And Chevron Report Lower Profits Amid Natural Gas Price Slump
Exxon and Chevron, two of the world's largest energy companies, recently released their financial reports, revealing low... Read more
Europe's Energy Independence: How It Solved The Russian Gas Problem
Europe's historical reliance on Russian gas has long been a central concern for its energy security. However, recent yea... Read more
Shifting Dynamics: How BHP's Bid For Anglo American Reshapes The Mining Sector
Market Dynamics Before the MergerImplications of the Proposed MergerRegional and Global Market EffectsCommodity-Specific... Read more