Machine Learning Applications In Carbon Credit Price Forecasting
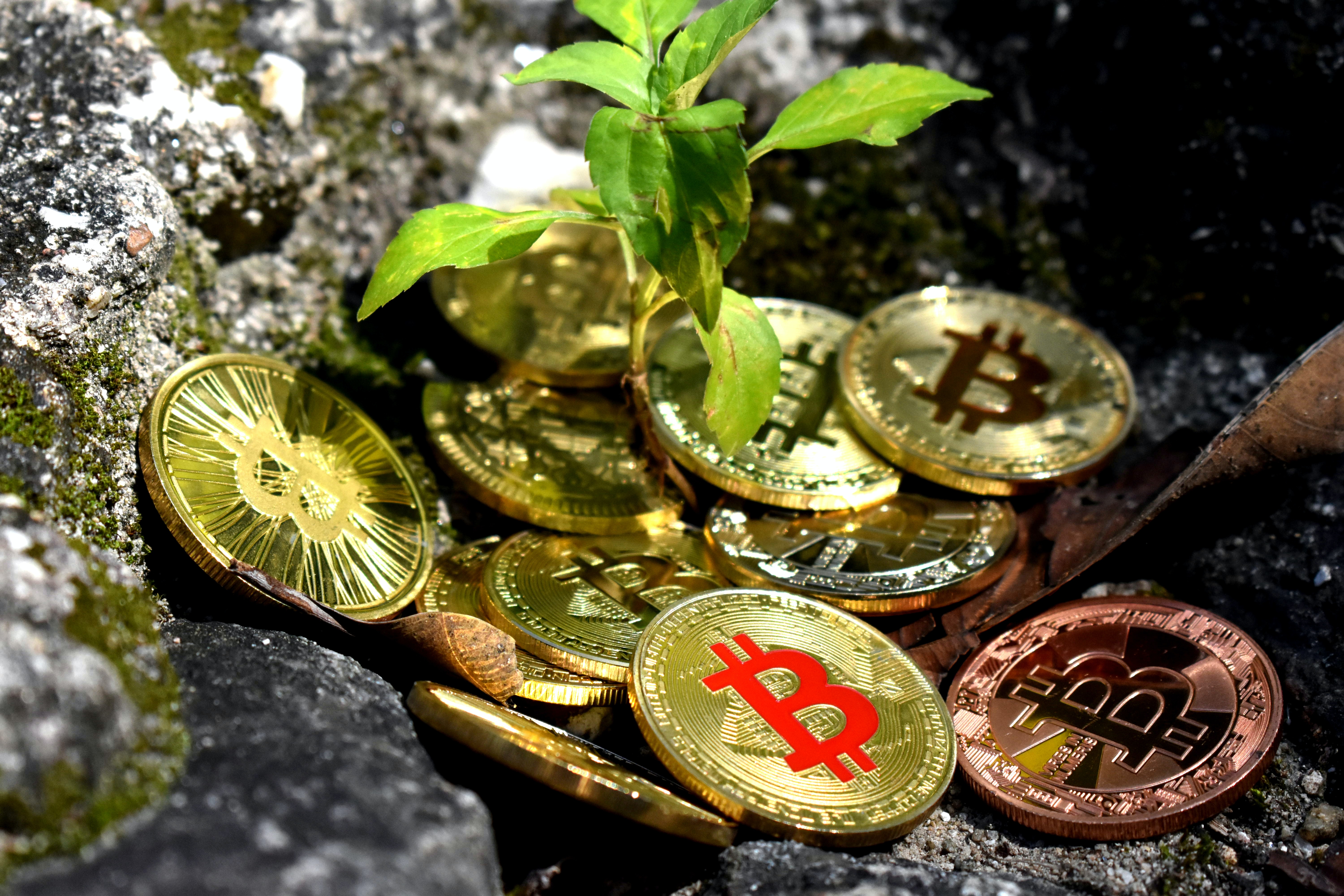
Machine learning applications in carbon credit price forecasting are revolutionizing the financial sector, particularly in green investments. A trending topic in sustainable finance, carbon credits refer to a permit or certificate enabling the holder to emit a certain amount of carbon dioxide or other greenhouse gases. Machine learning, on its side, dwells in the realm of artificial intelligence, and it’s generally recognized as a game changer in numerous industry fields including financial forecasting. This novel combination grants fascinating possibilities for accurate price prediction in the realm of green investments.
Digging into carbon credits, it's worthy to comprehend their functionality which, in fact, operates as a sort of environmental initiative designed to reduce emissions. Companies buy these credits, compensating for their carbon emissions and driving further investment in sustainable projects. The importance of accurate price forecasting in this sector underlines the fact that with more accurate predictions, firms and investors can craft strategic decisions that benefit both the environment and their balance sheets.
Linking machine learning to this forecasting puzzle resonates with its proven capabilities. Essentially, machine learning allows computers to learn and improve from experience without explicit programming. No surprise, these tools play an essential role in price forecasting, given its ability to process vast amounts of data and discern patterns humans might oversee. Translating this prowess into carbon credit price forecasts, means better predictions, propelling the industry towards more sustainable financial planning.
What can it look like when machine learning meets carbon credit price forecasting? Some key concepts to consider involve predictive analysis, data interpretation, and risk estimation. The fit between machine learning principles and carbon credit price prediction relies on a straightforwardly favourable proposition - accurate predictions lead to profitable decisions. Marrying these two domains hence equips financial entities with potent insights regarding the future price of carbon credits.
Considering the benefits of using machine learning in carbon credit pricing forecast, accuracy ranks as the star player. With more reliable predictions, stakeholders can make informed decisions, lowering risks associated with forecasting inaccuracies. Going beyond risk reduction, machine learning also allows for improved identification of market trends, further enhancing the strategic value of these predictions. By tapping into this cutting-edge technology, we empower companies to take full advantage of their green investments, harnessing ethical benefits and economic profit.
However, like any other technology, machine learning for carbon credit price forecast is not without flaws. Issues related to data quality and reliability remain evident. A robust model depends on the quality of data fed into it, so errors or inconsistencies can impact the results. Additionally, changing market conditions may mandate frequent model adjustments, creating an added layer of complexity.
It remains clear that machine learning holds immense potential in improving carbon credit price forecasting. An effective tool for sustainable finance, it combines the prowess of artificial intelligence and the promise of carbon credits. This alignment of technology and environmental cause is a bright spark in the economy, and it's anticipated that this application of machine learning could scale heights as yet unseen in the finance sector. In conclusion, whilst there are challenges to this integration, the benefits far outstrip them, making machine learning applications in carbon credit price forecasting an exciting frontier in sustainable finance.
Author: Gerardine Lucero
Copper's Comeback: Inside BHP And Lundin's Argentine Asset Acquisition
Copper, often dubbed "the metal of electrification," is experiencing a resurgence in demand due to its critical role in ... Read more
Revitalizing Commodities: How Clean Energy Is Breathing New Life Into A Stagnant Market
The commodities market, traditionally a cornerstone of investment portfolios, has experienced a decade of stagnation. Ho... Read more
European Airports Disrupted By Escalating Climate Protests
Climate activists have escalated their protests at European airports, blocking runways and causing flight disruptions in... Read more
Hungary's Russian Oil Dilemma: Why Brussels Is Cautious In Offering Support
Hungary's reliance on Russian oil has led it to seek support from Brussels to ensure continued access to this crucial en... Read more
Unveiling China's Secret Commodity Stockpiles: What Lies Ahead?
Xi Jinping's extensive reserves of grain, natural gas, and oil hint at future challenges.In a move shrouded in secrecy, ... Read more
Copper Miners Brace For Industry Overhaul As End Users Seek Direct Deals
The copper mining industry is bracing for a significant overhaul as end users, including cable manufacturers and car com... Read more